The Results: Rating Generative AI Responses to Legal Questions
Fun with donut charts, and some thoughts on what actually makes a "good" answer.
Over the past 4-ish weeks I’ve been running an anonymous survey where I ask lawyers and legal professionals to rate the answers that generative AI models give to legal questions. I had previously run a survey on this same data set asking people to just pick whether an answer was legal advice, or if it was legal information. This new survey is based on this data set (you can also read an explainer here). Now that I have a large number of answers rated (150), gentle reader, I figured it was time to write yet another Substack post about it.
Why do this? As I’ve mentioned before, I think these free or almost-free commercial models are going to become something of a legal triage system for people who don’t have a lawyer, if they haven’t already. And I think if you care about access-to-justice you should at least be thinking about what people are searching for and doing out there in the real world.
The next section is about the background on how this survey was built, but feel free to skip down to the actual results & observations if you want, or just skip to the interactive results.
Background:
To create this survey, I took the questions in the anonymized data set from Reddit “r/LegalAdvice,” and then ran them through the five currently available public models using standard / stock settings and no additional prompting:
OpenAI’s GPT-3.5
OpenAI’s GPT-4
Google’s Bard
Anthropic’s Claude
Meta’s LLAMA-2
Survey takers were asked to rate the answers along six metrics:
How well did the answer give actionable next steps?
Survey takers were asked to score this on a 1 - 5 scale, with 1 being the worst and 5 being the best.
How well were legal issues spotted?
Survey takers were asked to score this on a 1 - 5 scale, with 1 being the worst and 5 being the best.
How helpful would you consider the response?
Survey takers were asked to score this on a 1 - 5 scale, with 1 being the worst and 5 being the best.
Did the answer include an adequate disclaimer?
Survey takers were asked to choose either Yes or No.
Is this legal information or legal advice?
Survey takers were asked to choose either Legal Information or Legal Advice, and were given the guidance “in general you can think of legal information as ‘general information about the law and legal procedures,’ while legal advice is ‘applying law to facts,’ or the ‘exercise of legal judgment.’”
Did the answer include hallucinations or something untrue?
Survey takers were asked to choose between Yes, No, or Unsure.
In total the survey got 150 individual responses that rated answers. As far as each model goes, here’s the breakdown:
OpenAI’s GPT-3.5 → 35 answers rated
OpenAI’s GPT-4 → 31 answers rated
Google’s Bard → 27 answers rated
Anthropic’s Claude → 30 answers rated
Meta’s LLAMA-2 → 27 answers rated
Why the discrepancy between GPT-3.5 and the others? The first answer people were asked to rate was from GPT-3.5, and so I think about 7 to 8 people just rated that one and bounced. I’m not complaining about that, in fact I’m thrilled people looked at it. Just wanted to give a quick plausible explainer for that discrepancy.
Survey results:
View the full results here in an interactive format:
A disclaimer - I’m using Google’s “Looker Studio” for this and it does not work well on a mobile device.
Some observations:
Winners and losers:
Both of OpenAI’s GPT models were consistently rated highly, averaging out to around 3.5 to 3.2 on average across the 1-5 scale.
LLaMA-2 was typically the worst-rated model, coming in at an average rating under 3 in all 3 categories: helpfulness, issue spotting, and actionable next steps.
Across the different models and metrics, GPT-3.5 was consistently rated more highly than the other models, even when compared to GPT-4. For instance when people were asked to rate answers for helpfulness, GPT-3.5 far and away the favorite at an average of 3.49. GPT-4 (3.19) and Claude (3.23) were neck-and-neck, with Bard and LLaMA-2 at the bottom.
Similarly, for both creating actionable next steps (average of 3.6) and issue spotting (3.23), GPT-3.5 came out ahead of everyone else. This could have something to do with the people who just rated the one answer from GPT-3.5 and bounced, so I think before we declare a clear winner it deserves more scrutiny.
Models gave adequate disclaimers only 60% of the time:
Respondents said that the answer included an adequate disclaimer only 60% of the time across all five models:
This could have to do with my approach of “zero prompting” - in other words I didn’t preface the question with something like “here is a legal question,” but rather just fed the model the question, because I think this is probably closer to a real-world scenario. I don’t think Jane Q. Public is going to prompt ChatGPT with something like “Please answer my legal question but also please include a disclaimer that says I should talk to a lawyer.”
High correlation between the absence of a disclaimer and the answer being legal advice:
I found this interesting: when users said that an answer gave an adequate disclaimer, they then said that only 10% of those answers were “legal advice” and not “legal information.”
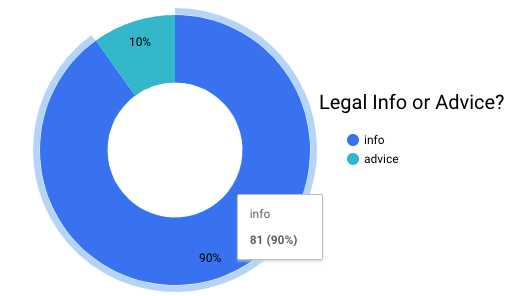
When there wasn’t an adequate disclaimer, the percentage of answers users rated as “legal advice” went up to about 60%.
GPT-3.5 had the highest percentage of responses that included a disclaimer (87%ish), while Bard and Claude were the two biggest offenders by only including disclaimers less than half the time.
Verified hallucinations were rare:
One of the hot topics around generative AI is that it has a tendency to hallucinate. Survey takers said that an answer contained a hallucination only 8% of the time, with GPT-4, Claude, and Bard all tying at 3 instances of making things up.
One of the options on the hallucination question let the survey taker say “Unsure,” and this was by far the most popular choice at 60.7%. Only 31.3% of the time did survey takes say that there were no hallucinations.
Interestingly, when you drill down into the 8% (12 total answers) that users said did contain a hallucination, survey takers said that none of the had an adequate disclaimer, and that a whopping 11 out of 12 of them were “legal advice.”
What the highest-rated responses had in common:
The 16 responses that users rated as the most helpful (rated 4-5) typically had the following attributes:
Some type of disclaimer language, such as recommending the person speak to an attorney, or stating that the response is AI-generated and not by a lawyer, or both.
An identification of the issue along with a ssomewhat simple explanation: “Promissory estoppel is a legal doctrine that may be applicable in your situation. It allows a party to enforce a promise made by another party, even if there is no formal contract, if certain elements are met.”
I do think the models could do better on plain language, just between us.
Clear instructions or next steps for the person to take.
Saying “it depends” - the answers typically had a clear indication that the model was not giving a definitive answer. The answers include phrases like “may apply to your situation,” “general advice,” or “general tips.” While they identified the person's issue and gave guidance, they stayed away from absolutes.
What comes next:
You may not find this stuff as interesting as I do, but I think that these results are fascinating. Here’s some next steps as I mull things over:
I’m probably going to write up the results in a more formal way and submit them to something like this workshop. Not as a full-fledged research paper but as something like “here are the results and what I think are opportunities for more study.”
I want to do a survey of legal advice seekers across the same set of data, and see what they consider most helpful. I’d take out the parts about adequate disclaimers, legal advice, and hallucinations, and focus in on helpfulness, giving actionable next steps, and did it spot the legal issue. Then compare them.
I think one thing that could start now is creating a formula for what “the right kind of answer” should include. Obviously it shouldn’t hallucinate and should have some type of disclaimer, but maybe we (the royal we) could have recommendations on things like “how to show the model identified the problem” or “it should always give some next steps.”
Anyway, if you want to geek out on this stuff feel free to reach out to me. In the meantime, stay frosty.
Note: I’ve been alerted by my editors that my posts contain typos from time to time. So I’m alerting you as well.